Labeling Tools for Machine Learning: Unlocking the Power of Data Annotation
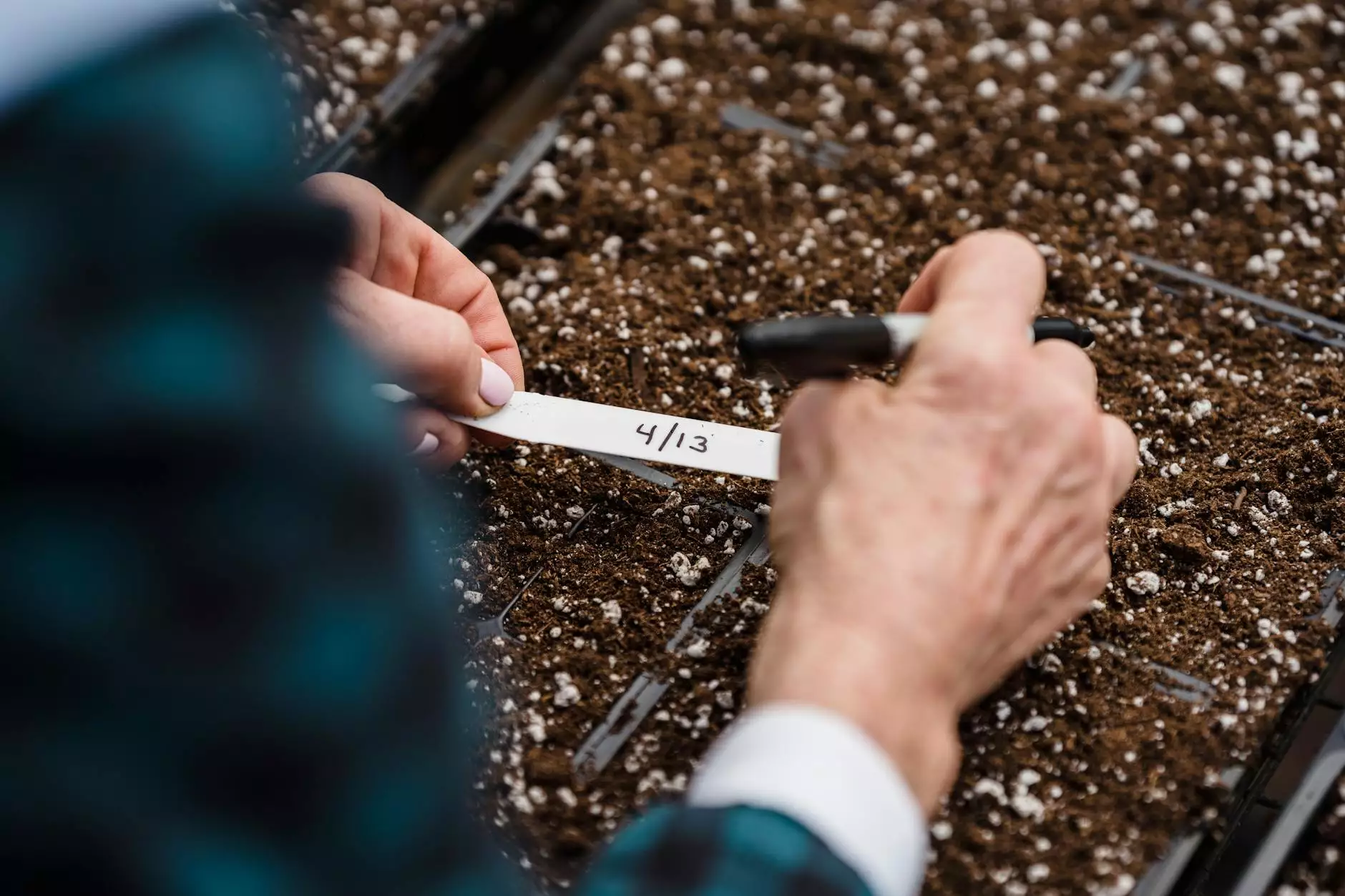
The landscape of machine learning is continually evolving, with the demand for high-quality data growing at an unprecedented rate. At the heart of developing effective AI models lies a critical process: data annotation. This process involves labeling data so that machine learning algorithms can learn from it. In this guide, we will delve deep into the world of labeling tools for machine learning, exploring their significance, functionalities, and how they can streamline your data annotation efforts.
Understanding Data Annotation and Its Importance
Before diving into the specifics of labeling tools, it’s essential to comprehend what data annotation entails and why it is vital in machine learning.
- Data Annotation Defined: Data annotation refers to the process of tagging or labeling data to make it understandable for machine learning algorithms. This can include image tagging, text classification, signal processing, and more.
- Importance of Data Annotation: Accurate data annotation is crucial because machine learning models learn from labeled data. Poorly annotated data can lead to inaccurate models, resulting in ineffective outcomes.
- Application in Various Fields: Data annotation serves as the foundation for several industries, including healthcare, automotive (autonomous vehicles), e-commerce (product recommendation systems), and finance (fraud detection).
Types of Labeling Tools for Machine Learning
Several types of labeling tools for machine learning cater to different data types and project requirements. Each tool is designed to facilitate specific forms of data annotation.
Text Annotation Tools
Text annotation tools help label various textual data types such as documents, articles, and social media content. Some common functionalities include:
- Entity Recognition: Identify and label entities within text such as names, organizations, and locations.
- Sentiment Analysis: Label text data to denote sentiments, which is valuable for understanding customer opinions and market feedback.
- Intent Recognition: Determine the intent behind user queries, enhancing conversational AI applications.
Image and Video Annotation Tools
For computer vision applications, image and video annotation tools are paramount. These tools offer various features, such as:
- Bounding Box Annotation: Draw bounding boxes around objects in images for detection tasks.
- Semantic Segmentation: Label each pixel of an image, providing detailed information for tasks like image recognition.
- Keyframe Annotation: Annotate specific frames in a video to track object movements and behaviors.
Audio Annotation Tools
Audio annotation tools are designed to manage audio signals for applications like speech recognition and sound classification. Key functionalities include:
- Transcription: Convert spoken language into text format, perfect for creating datasets for voice assistant technologies.
- Sound Event Detection: Label occurrences of specific sounds for environmental sound recognition.
Key Features of Effective Labeling Tools
When selecting labeling tools for machine learning, certain features should be prioritized to ensure efficiency and accuracy.
User-Friendly Interface
An intuitive interface reduces the learning curve for users, making it easier for annotators to conduct their work effectively. The best tools offer:
- Drag-and-Drop Functionality: Simplifies the process of uploading and annotating data.
- Collaborative Features: Allows multiple users to work on projects simultaneously, enhancing productivity.
Integration Capabilities
The ability to integrate seamlessly with other software and platforms is vital. Ideal labeling tools should allow for:
- API Access: Facilitate interactions with machine learning models and other databases.
- Data Export Options: Support various formats so annotated data can be easily utilized in different applications.
Quality Control Mechanisms
Maintaining high-quality annotations is essential. Tools should incorporate:
- Automated Quality Assurance Checks: Help identify labeling errors before finalizing datasets.
- Reviewer Roles: Assign roles for quality checkers to validate and correct annotations made by others.
The Benefits of Using Labeling Tools for Machine Learning
Utilizing dedicated labeling tools offers significant advantages that can dramatically improve project outcomes.
Increased Efficiency
Manual annotation can be time-consuming and prone to human error. By implementing advanced labeling tools, organizations can:
- Accelerate Annotation Processes: Speed up data preparation time, allowing for faster model training.
- Reduce Costs: Minimize labor costs by leveraging automation features available in modern labeling tools.
Improved Labeling Accuracy
Accuracy is paramount in machine learning. High-quality labeling tools ensure:
- Consistency: Standardized labeling processes lead to consistent datasets.
- Manual Review Options: Opportunities for human verification enhance the reliability of annotations.
Scalability
As data volumes grow, so does the need for scalable annotation solutions. Effective labeling tools provide:
- Handling Large Datasets: Capable of processing extensive datasets without performance degradation.
- Flexible Project Management: Efficiently manage multiple projects with varying requirements simultaneously.
Choosing the Right Labeling Tool: A Comprehensive Criterion
For businesses looking to invest in labeling tools, it’s essential to consider several criteria to find the best fit.
Determine Your Needs
Different projects require different types of annotations. Begin by assessing:
- The Nature of Your Data: Is it predominantly text, images, audio, or a combination?
- Your Team’s Skill Set: Is your team familiar with specific tools, or do they require training?
Evaluate Pricing Models
Look for tools that align with your budget while offering the necessary functionalities. Consider:
- One-Time Payment vs. Subscription: Decide between a significant upfront investment or ongoing monthly costs.
- Cost per Annotation: Some platforms charge based on the number of annotations made, which can scale as your project expands.
Trial and Feedback Mechanisms
Most reputable tools offer trials. Take advantage of these by:
- Conducting Pilot Projects: Test the tool with a small dataset to evaluate its performance.
- Gathering Team Feedback: Collect input from your annotators on usability and features.
Conclusion: Harnessing the Potential of Labeling Tools for Machine Learning
In conclusion, utilizing labeling tools for machine learning is crucial in today’s data-driven world. With the right tools, organizations can significantly enhance their data annotation efficiency, accuracy, and scalability. Whether you operate in e-commerce, healthcare, or automotive sectors, choosing effective labeling tools like those offered by KeyLabs.ai will empower you to build robust AI models, unlocking new possibilities in your business.
Remember, the quality of your machine learning models directly correlates with the quality of your data annotations. Invest wisely in labeling tools, and elevate your machine learning initiatives to unprecedented heights.